Methods for Constraining Climate Projections (CMIP6) over Canada to Support the Hydrological Community
This project aims to reduce snow-related uncertainty in pan-Canadian projections by leveraging the latest-generation CMIP6 GCM projection data. The research involves the use of a weighting method called ClimWIP to reduce the uncertainty of CMIP6 GCMs based on their performance, with a particular focus on accurately reproducing the annual maximum of the water equivalent of snow.
Project details
Principal(s) investigator(s)
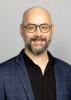
Context
In winter, precipitation mainly accumulates in the form of snow or ice. With the melting of the snow in the spring, large quantities of water flow into our waterways, which can often lead to flooding.
In Canada, the most common floods are those resulting from snowmelt runoff. This is of particularly concern due to the great uncertainty related to snow in the context of climate change.
Objective(s)
This project has three objectives :
-
To outline the most recent advances in emergent constraints, through a literature review and personal communications, in order to select the method(s) to be applied
-
To reduce the uncertainty of climate projections by applying emergent constraints tailored to Canada
-
To enable an informed choice of GCMs for driving data, representing the uncertainty spectrum while selecting the most plausible climate trajectories
Methodology
Apply the concepts of emergent constraints, i.e., find a relationship between one or more x variables in current climate model simulations and a different y variable in future climate model projections. To do this, the performance of the simulated variables must be applied.
Results
Based on performance and independence, we selected one of the emergent constraint methods provided by ESMvaltool.org that had been explored.
This method allowed us to identify the most effective predictors for reproducing the annual maximum of the water equivalent of snow:
-
One of them was the daily climatology of the surface level temperature, precipitation and sea level pressure using bandpass filtering for 2 to 6 days;
-
Another was the climatology of annual snowfall peaks.
Moreover, the analysis shows that the main effect of the weighting process is consistent in the past and future absolute fields, with minimal impact on climate change patterns. Spatial averaging shows that the weighted and unweighted anomalies are not significantly different, although we observed a weaker upper percentile for surface level temperature and precipitation climatology in the weighted results.
In addition, our weighted results were compared to various filtering approaches, including the “one model, one vote” and “one family, one vote” approach as in Merrifield et al. (2020), “one institution, one vote” as in Kuma et al. (2023), and the equilibrium climate sensitivities ranges suggested by Hausfather et al. (2022).
This comparative analysis highlights the effectiveness of the ClimWIP approach, with a focus on the influence of performance-based weighting on regional climate variables. The analysis of the CMIP6 models clearly showed that certain models are more suitable for use in Canada.
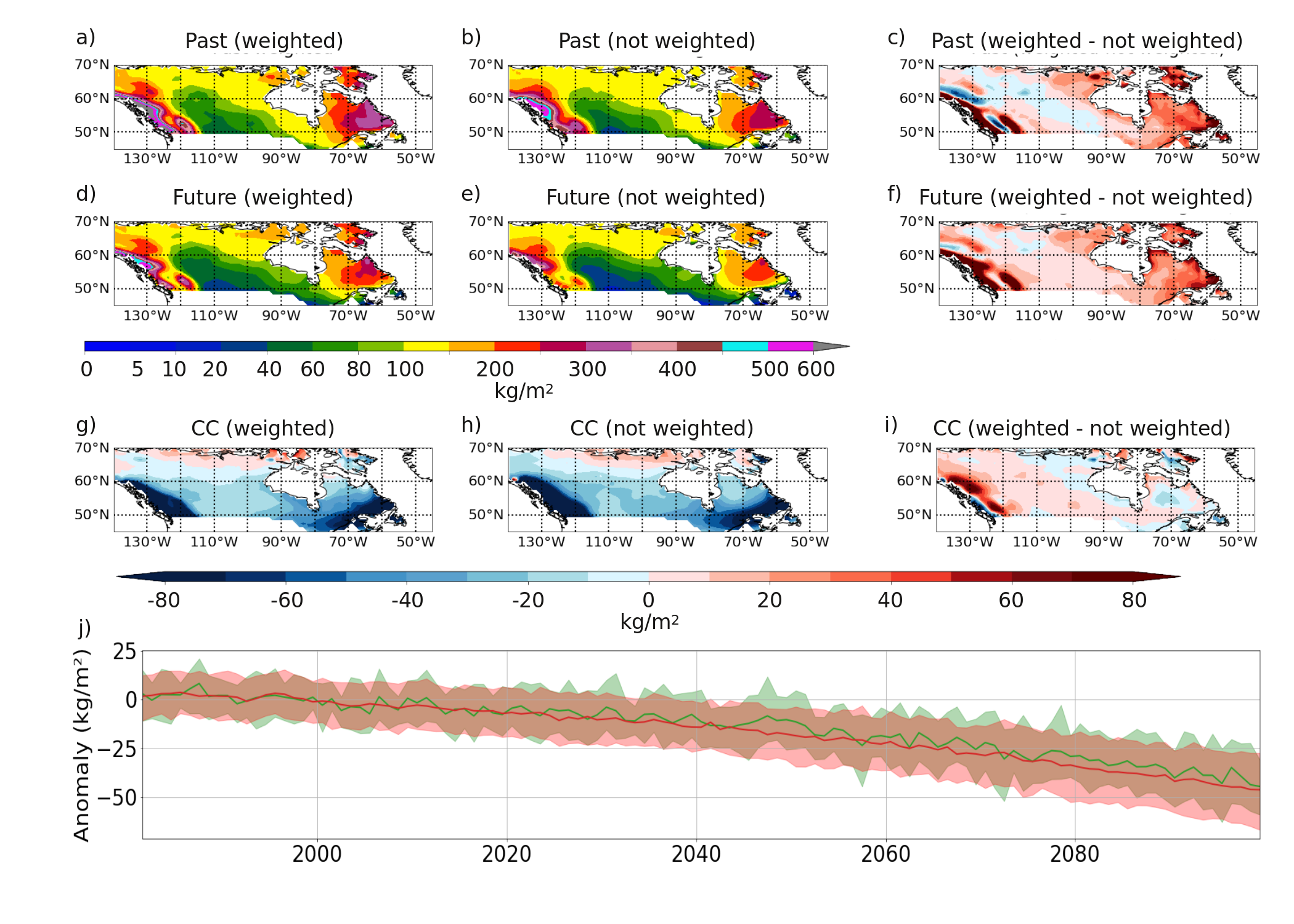
Figure 1: Fig. 1: Weighted and unweighted results for snow water equivalent climatology, shown in the left and right panels, respectively. Panels (a), (d) and (g) illustrate the weighted climatology for the past (1981-2010), the future and climate change, while panels (b), (e) and (h) show the unweighted versions. The differences between the weighted and unweighted results for the past, the future and climate change are illustrated in panels (c), (f) and (i), respectively. Panel (j) shows the changes in the mean spatial anomaly, with the thick red line representing the unweighted dataset and the green line representing the weighted dataset in the interval of 16.6 to 83.4 percentiles. Matte, Leduc, Labonté et Paquin, in prep.
Benefits for adaptation
This project’s methods and analyses may guide certain decisions for the configuration of climate datasets for other research projects.
This innovation can contribute to any study that uses datasets and may answer some of the research questions on good weighting practices. These practices are already used in hydrology and can be extended to other sectors such as agriculture, health, infrastructure or adaptation, to name a few.
Funding
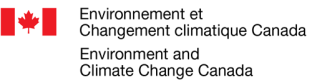
Other participants
- Dominique Paquin, Ouranos
- Marie-Pier Labonté, Ouranos
- Martin Leduc, Ouranos
- Isabelle Chouinard, Ouranos
- John Scinnoca, Centre canadien de la modélisation et de l'analyse climatique
Related projects
710400